24th UK Conference on
Medical Image Understanding and Analysis
Conference materials are available on YouTube now
COVID-19 Update
Due to growing concerns about the COVID-19 epidemic, we have regrettably decided to cancel the physical meeting this year and move MIUA 2020 to a virtual context.
We appreciate the work that has gone into preparing contributions, hence we are still welcoming paper submissions until 3rd April using our online submission system. Please note that this deadline is final as no further extensions can be granted at this point. All submissions will be peer-reviewed and accepted articles will be published as MIUA Proceedings by the Springer Publishing Group. Authors of accepted papers will be invited to upload a video of their presentations, which all registered attendees will have access to during the conference dates (15th - 17th July 2020). Details on registration for this new format (including registration fees to cover costs) will be shared shortly.
Thank you for your understanding, and we look forward to delivering MIUA 2020 in a way that is rewarding for our authors and interesting for the research community as a whole.
Furthermore, we are excited to announce that following this digital meeting, we hope to welcome you in person for MIUA 2021 next year which will be held in Oxford on 12th - 15th July 2021.
Scope
MIUA is a UK-based international conference for the communication of image processing and analysis research and its application to medical imaging and biomedicine. This is a rapidly growing subject with ever increasing real-world applicability.
MIUA welcomes all researchers in medical imaging including mathematicians, computer scientists, bioinformaticians, clinicians, engineers and bioscientists.
MIUA is the principal UK forum for communicating research progress within the community interested in image analysis applied to medicine and related biological science. The meeting is designed for the dissemination and discussion of research in medical image understanding and analysis, and aims to encourage the growth and raise the profile of this multi-disciplinary field by bringing together the various communities including among others:
Brain imaging | Cancer | Cardiac Imaging | Circulation and Microcirculation |
Computational anatomy and physiology | Computed Tomography | Dermatology | Imaging Physics |
In-vivo intravital imaging | Inflammation | Magnetic Resonance Imaging | Microscopy | Neurology | Novel Imaging Methods | Ophthalmology | Optical Imaging |
Positron Emission Imaging | Radiology | Tissue Perfusion | Ultrasound |
Keynote Speakers
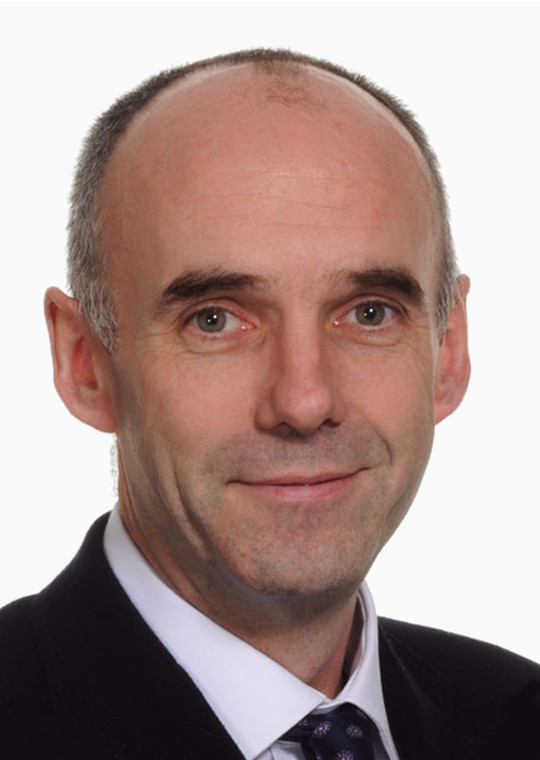
Fergus Gleeson
Consultant Radiologist and Professor of Radiology, Oxford.
Prof. Fergus Gleeson
Consultant Radiologist and Professor of Radiology, University of Oxford
Artificial Intelligence: a clinical perspective of the challenges and opportunities [video]
Biography: Professor Fergus Gleeson is a Consultant Radiologist and Professor of Radiology in Oxford. He trained in Cambridge, Papworth and London, and was a Fellow in Radiology at UCLA in America. He was appointed to Oxford in 1992, is Head of Academic Radiology in Oxford, running the ACF and PhD programme, and is the Director of the Oxford Radiology Research Unit at Oxford University Hospitals NHS Foundation Trust. He has published over 200 peer review papers and book chapters, and has more than £20 million in grant income. His specialist interests are in Artificial Intelligence, Thoracic Imaging, PET-CT and Hyperpolarized xenon MRI. Fergus is also the Chief Medical Officer of the National Consortium of Intelligent Medical Imaging (NCIMI): which aims to bring together the NHS, and University and industry partners to promote the development and implementation of artificial intelligence in medicine.
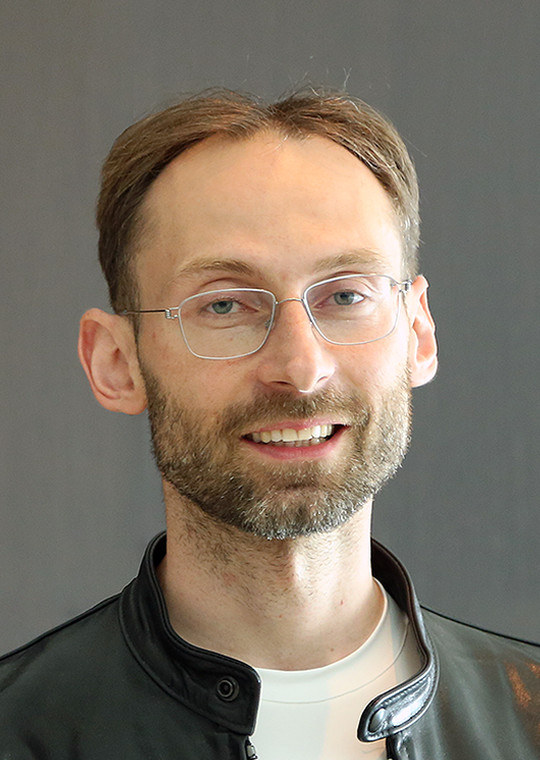
Michael Bronstein
Chair in Machine Learning and Pattern Recognition, Imperial College London.
Prof. Michael Bronstein
Chair in Machine Learning and Pattern Recognition, Imperial College London Head of Graph Learning Research, Twitter
Geometric deep learning on graphs, manifolds, and beyond [video]
Biography: Michael Bronstein is a professor at Imperial College London, where he holds the Chair in Machine Learning and Pattern Recognition, and Head of Graph Learning Research at Twitter. His main research expertise is in theoretical and computational methods for geometric data analysis, a field in which he has published extensively in the leading journals and conferences. He is credited as one of the pioneers of geometric deep learning, generalising machine learning methods to graph-structured data. Michael received his PhD from the Technion (Israel Institute of Technology) in 2007. He has held visiting appointments at Stanford, MIT, Harvard, and Tel Aviv University, and has also been affiliated with three Institutes for Advanced Study (at TU Munich as Rudolf Diesel Fellow (2017-), at Harvard as Radcliffe fellow (2017-2018), and at Princeton (2020)). Michael is the recipient of five ERC grants, Fellow of IEEE and IAPR, ACM Distinguished Speaker, and World Economic Forum Young Scientist. In addition to his academic career, Michael is a serial entrepreneur and founder of multiple startup companies, including Novafora, Invision (acquired by Intel in 2012), Videocites, and Fabula AI (acquired by Twitter in 2019). He has previously served as Principal Engineer at Intel Perceptual Computing and was a key contributor to the RealSense 3D sensing technology.
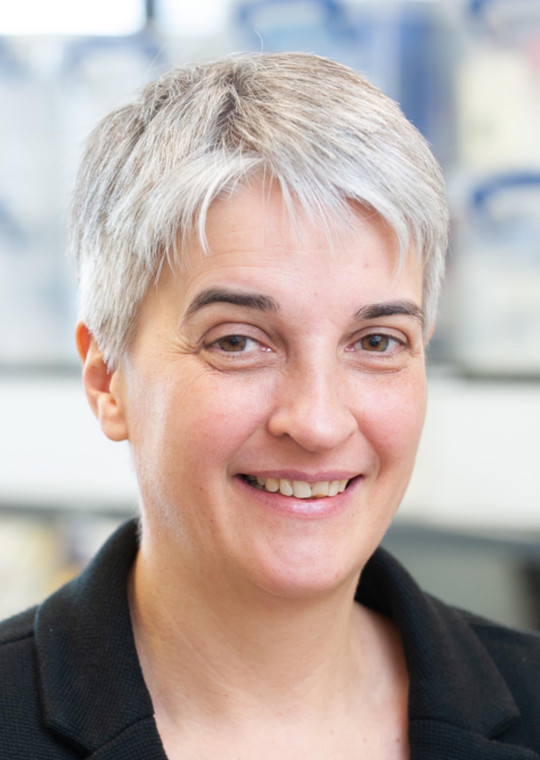
Julia Schnabel
Chair in Computational Imaging, King's College London.
Prof. Julia Schnabel
Chair in Computational Imaging, King's College London
Smart Medical Imaging -- from medical image acquisition to analysis and understanding [video]
Abstract: Machine learning, and in particular deep learning approaches in medical imaging have shown great promise in the areas of disease detection, classification and image segmentation, and are now moving into more complex topics such as motion correction and shape modelling. However, their success is limited by the availability and quality of the images in the dataset used for training these algorithms. A common approach is to train deep learning methods on well annotated and curated databases of high-quality image acquisitions, which then may fail on real patient cases in a hospital setting. Another problematic is the lack of sufficient numbers of clinical label annotations in the training data. In this talk I will present some of our recent deep learning approaches that aim to address these two challenges, by applying novel methods for realistic data augmentation and image quality control. To illustrate these approaches, I will draw from our examples in cardiac magnetic resonance imaging, and fetal/maternal ultrasound. I will conclude by giving an outlook on the future opportunities in this field, operating from the imaging sensor right through to extracting clinically relevant measures.
Biography: Julia Schnabel was appointed Chair in Computational Imaging at the School of Biomedical Engineering and Imaging Sciences, King’s College London in 2015. She previously was Professor of Engineering Science (Medical Imaging) at the University of Oxford, in association with a Tutorial Fellowship in Engineering at St. Hilda’s College, Oxford. She holds an MSc in Computer Science (Dipl. Inform.) from the Technical University of Berlin, and a PhD in Computer Science from University College London, University of London. After her PhD she held post-doctoral positions at University College London, King’s College London and University Medical Center Utrecht. Her research is focusing on machine/deep learning, complex motion modelling, as well as multi-modality and quantitative imaging, with applications to cancer, cardiac and fetal imaging. Since 2007 Julia has supervised over 50 PhD students and Postdocs, and is the Director of the EPSRC Centre for Doctoral Training in Smart Medical Imaging, run jointly between King’s College London and Imperial College London. She is an Associate Editor of IEEE Transactions on Medical Imaging, of IEEE Transactions on Biomedical Engineering, on the Editorial Board of Medical Image Analysis, and has recently founded a new free open access journal of Machine Learning for Biomedical Imaging (melba-journal.org) which will be launched in summer 2020. She was a Director of the Medical Imaging Summer School (MISS) on the topics of Computer Vision (2014), Machine Learning (2016) and Deep Learning (2018), Program Chair of the Medical Image Computing and Computer Assisted Intervention (MICCAI) conference (2018), General Chair of the International Workshop in Biomedical Image Registration (WBIR) (2016), and will be a General Chair of the Information Processing in Medical Imaging (IPMI) conference to be held in 2021. She is serving on the IEEE EMBS Administrative Committee and the MICCAI Society Board of Directors. In 2018, Julia was elected Fellow of the MICCAI Society “For contributions to multiple areas of medical image computing, and for distinguished service to the MICCAI conference and Society”, and in 2019 she became Fellow of the European Laboratory for Learning and Intelligent Systems (ELLIS) as a member of ELLIS Health.
Important Dates
Submission System Opens | 15th January 2020 |
Paper Submission Deadline | |
Registration Open | April 2020 |
Author Notification | 4th May 2020 |
Camera-Ready Submission Deadline | 11th May 2020 |
Virtual Conference | 15th - 17th July 2020 |
Call for Papers
You are invited to submit your full paper to MIUA 2020 which will be held in Oxford, UK. The paper submission system will open on 15th January 2020.
The full paper submission deadline will be 23:59, Greenwich Mean Time (GMT), on 3rd April 2020.
High-quality papers are requested, containing original contributions to the topics within the scope of MIUA. Submitted full papers should be formatted in the CCIS style, consisting a maximum of 12 pages (incl. text, figures, tables, references). Accepted papers will be included in the conference proceedings published by Springer. Authors should consult Springer’s authors’ guidelines and use their proceedings templates, either for LaTeX or for Word, or Overleaf for the preparation of their papers. Submissions are to be made to the CMT system, and the review process will be double-blind.
Springer encourages authors to include their ORCIDs in their papers. In addition, the corresponding author of each paper, acting on behalf of all of the authors of that paper, must complete and sign a Consent-to-Publish form. The corresponding author signing the copyright form should match the corresponding author marked on the paper. Once the files have been sent to Springer, changes relating to the authorship of the papers cannot be made.
MIUA continues to foster fairness, diversity, and inclusion within its community. Submissions from typically underrepresented groups are particularly encouraged.
Conference Programme
All accepted papers will be published as a volume in Springer Proceedings. The video presentations will be made available to registered attendees.
Image Segmentation
Textural feature based segmentation approach: A repeatable and accurate segmentation for tumors in PET images [video] [pdf] Elisabeth Pfaehler, Liesbet Mesotten, Gem Kramer, Michiel Thomeer, Karolien Vanhove, Johan de Jong, Peter Adriaensens, Otto S. Hoekstra, Ronald Boellaard | |
Unlearning Scanner Bias for MRI Harmonisation in Medical Image Segmentation [video] [pdf] Nicola K. Dinsdale, Mark Jenkinson, Ana I. L. Namburete | |
Semantic Segmentation of Histopathological Slides for the Classification of Cutaneous Lymphoma and Eczema [video] [pdf] Jeremy Scheurer, Claudio Ferrari, Luis G. Berenguer Todo-Bom, Michaela Beer, Werner Kempf, Luis Haug | |
Autofocus Net: Auto-focused 3D CNN for Brain Tumour Segmentation [video] [pdf] Andreas Stefani, Roushanak Rahmat, David Harris-Birtill | |
Cortical Plate Segmentation using CNNs in 3D Fetal Ultrasound [video] [pdf] Madeleine K. Wyburd, Mark Jenkinson, Ana I. L. Namburete | |
Improving U-Net Segmentation with Active Contour Based Label Correction [video] [pdf] Linde S. Hesse, Ana I. L. Namburete | |
Segmenting Hepatocellular Carcinoma in Multi-Phase CT [video] [pdf] Nora Vogt, Michael Brady, Gerard Ridgway, John Connell, Ana I. L. Namburete | |
On New Convolutional Neural Network Based Algorithms for Selective Segmentation of Images [video] [pdf] Liam Burrows, Ke Chen, Francesco Torella | |
Segmentation of the biliary tree from MRCP images via the monogenic signal [video] [pdf] George P. Ralli, Gerard R. Ridgway, Michael Brady | |
Transfer Learning for Brain Segmentation: Pre-task Selection and Data Limitations [video] [pdf] Jack Weatheritt, Daniel Rueckert, Robin Wolz | |
Pancreas segmentation-derived biomarkers: volume and shape metrics in the UK Biobank imaging study [video] [pdf] Alexandre Triay Bagur, Gerard Ridgway, John McGonigle, Michael Brady, Daniel Bulte | |
Localization and Identification of Lumbar Intervertebral Discs on Spine MR Images with Faster RCNN Based Shortest Path Algorithm [video] [pdf] Merve Zeybel, Yusuf Sinan Akgül | |
DeepSplit: Segmentation of Microscopy Images Using Multi-Task Convolutional Networks [video] [pdf]] Andrew Torr, Doga Basaran, Julia Sero, Jens Rittscher, Heba Z. Sailem |
Image Registration, Reconstruction, and Enhancement
A framework for jointly assessing and reducing imaging artefacts automatically using texture analysis and total variation optimisation for improving perivascular spaces quantification in brain magnetic resonance imaging [video] [pdf] Jose Bernal, María del C. Valdés-Hernández, Lucia Ballerini, Javier Escudero, Angela C. C. Jochems, Una Clancy, Fergus N. Doubal, Michael S. Stringer, Michael J. Thrippleton, Rhian M. Touyz, Joanna M. Wardlaw | |
Groupwise Multimodal Image Registration using Joint Total Variation [video] [pdf] Mikael Brudfors, Yaël Balbastre, John Ashburner | |
CT Scan Registration with 3D Dense Motion Field Estimation Using LSGAN [video] [pdf] Essa R. Anas, Ahmed Onsy, Bogdan J. Matuszewski | |
A Supervised Image Registration Approach for Late Gadolinium Enhanced MRI and Cine Cardiac MRI using Convolutional Neural [video] [pdf] Roshan Reddy Upendra, Richard Simon, Cristian A. Linte | |
Unsupervised Deep Learning for Stain Separation and Artifact Detection In Histopathology Images [video] [pdf] Andrew Moyes, Kun Zhang, Ming Ji, Huiyu Zhou, Danny Crookes | |
Asymmetric Point Spread Function Estimation and Deconvolution for Serial-Sectioning Block-Face Imaging [video] [pdf] Claire L. Walsh, Natalie Holroyd, Rebecca Shipley, Simon Walker-Samuel |
Ocular Imaging
A Deep Learning Approach for Semantic Segmentation of Gonioscopic Images to Support Glaucoma Categorization [video] [pdf] Andrea Peroni, Carlo A. Cutolo, Luis A. Pinto, Anna Paviotti, Mauro Campigotto, Caroline Cobb, Jacintha Gong, Sirjhun Patel, Andrew Tatham, Stewart Gillan, Emanuele Trucco | |
A Deep Learning Approach to Detect the Demarcation Line in OCT Images [video] [pdf] Chadi Helwe, Shady Elbassuoni, Ahmad Dhaini, Lily Chacra, Shady Awwad | |
Retinal Biomarkers Discovery for Cerebral Small Vessel Disease in an Older Population [video] [pdf] Lucia Ballerini, Ahmed E. Fetit, Stephan Wunderlich, Ruggiero Lovreglio, Sarah McGrory, María del C. Valdés-Hernández, Tom J. MacGillivray, Fergus Doubal, Ian J. Deary, Joanna Wardlaw, Emanuele Trucco | |
Simultaneous Optimisation of Confocal and Non-Confocal Images in an AOSLO with a Reconfigurable Aperture Pattern [video] [pdf] Biswajit Pathak, Laura Young, Hannah Smithson |
Radiomics, Predictive Models, and Quantitative Imaging Biomarkers
Automatic and Objective Facial Palsy Grading Index Prediction using Deep Feature Regression [video] [pdf] Anish Raj, Oliver Mothes, Sven Sickert, Gerd Fabian Volk, Orlando Guntinas-Lichius, Joachim Denzler | |
Prediction of Thrombectomy Functional Outcomes using Multimodal Data [video] [pdf] Zeynel A. Samak, Philip Clatworthy, Majid Mirmehdi | |
Radiomics: a New Biomedical Workflow to Create a Predictive Model [video] [pdf] Albert Comelli, Alessandro Stefano, Claudia Coronnello, Giorgio Russo, Federica Vernuccio, Roberto Cannella, Giuseppe Selvaggio, Roberto Lagalla, Stefano Barone | |
Ridge Detection and Analysis of Susceptibility-Weighted Magnetic Resonance Imaging in Neonatal Hypoxic-Ischaemic Encephalopathy [video] [pdf] Zhen Tang, Sasan Mahmoodi, Srinandan Dasmahapatra, Angela Darekar, Brigitte Vollmer | |
A machine learning approach for Colles’ fracture treatment diagnosis [video] [pdf] Kwun Ho Ngan, Artur d’Avila Garcez, Karen M. Knapp, Andy Appelboam, Constantino Carlos Reyes-Aldasoro | |
Investigating sex related phenotype changes in knockout mice by applying deep learning to X-ray images [video] [pdf] Kolawole Babalola, Hamed Haseli Mashhadi, Violeta Muñoz-Fuentes, Jeremy Mason, Terry Meehan, and On behalf of the International Mouse Phenotyping Consortium | |
Going Deeper into Cardiac Motion Analysis to Model Fine Spatio-Temporal Features [video] [pdf] Ping Lu, Huaqi Qiu, Chen Qin, Wenjia Bai, Daniel Rueckert, J. Alison Noble | |
A Lightweight CNN and Joint Shape-Joint Space (JSˆ2) Descriptor for Radiological Osteoarthritis Detection [video] [pdf] Neslihan Bayramoglu, Miika T. Nieminen, Simo Saarakkala | |
Discovering Unknown Diseases with Explainable Automated Medical Imaging [video] [pdf] Claire Tang |
Biomedical Simulation and Modelling
Deep Generative Models to Simulate 2D Patient-Specific Ultrasound Images in Real Time [video] [pdf] Cesare Magnetti, Veronika A. Zimmer, Nooshin Ghavami, Emily Skelton, Jacqueline Matthew, Karen Lloyd, Jo Hajnal, Julia A. Schnabel, Alberto Gomez | |
Volumetric Simulation of Nano-Fibres and 2D SEM and 3D XCT Imaging Processes [video] [pdf] John P. Chiverton, Alexander Kao, Marta Roldo, Gianluca Tozzi |
Mathworks Workshop
Dr Julia Hoerner
Deep Learning Academic Liaison Manager EMEA
Workshop on Deep Learning for Brain Images [video]
MathWorks engineers will demonstrate the latest MATLAB Deep Learning tools for medical image analysis.
Best Paper Awards
Our Sponsors
We gratefully acknowledge the generous support provided by the following patrons:
Silver
Bronze+
Bronze
Organizing Committee
The Organizing Committee is comprised of academic members from the Medical Sciences Division (Nuffield Department of Clinical Neurosciences [NDCN], and The Big Data Institute [BDI]) and the Mathematical Physical and Life Sciences Division (Institute of Biomedical Engineering [IBME]), representing Oxford’s core strategic partners in medical imaging research.
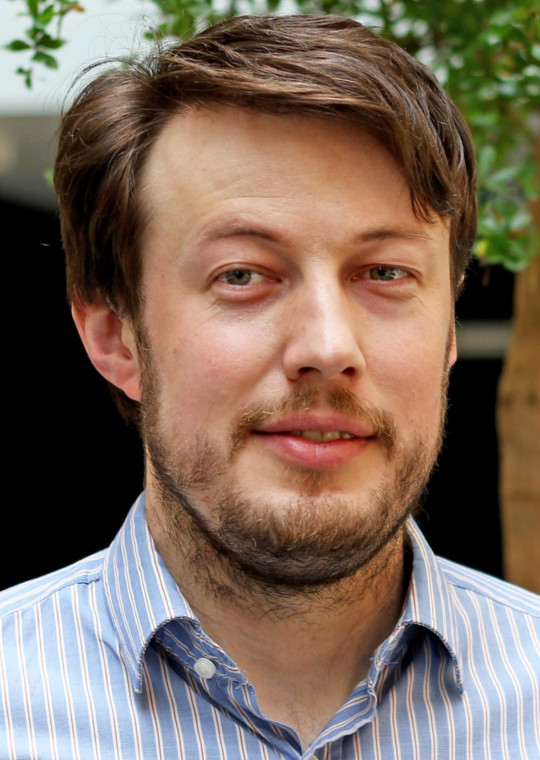
Bartlomiej Papiez
Research Fellow at the Big Data Institute.
Bartlomiej Papiez
BDI, Oxford
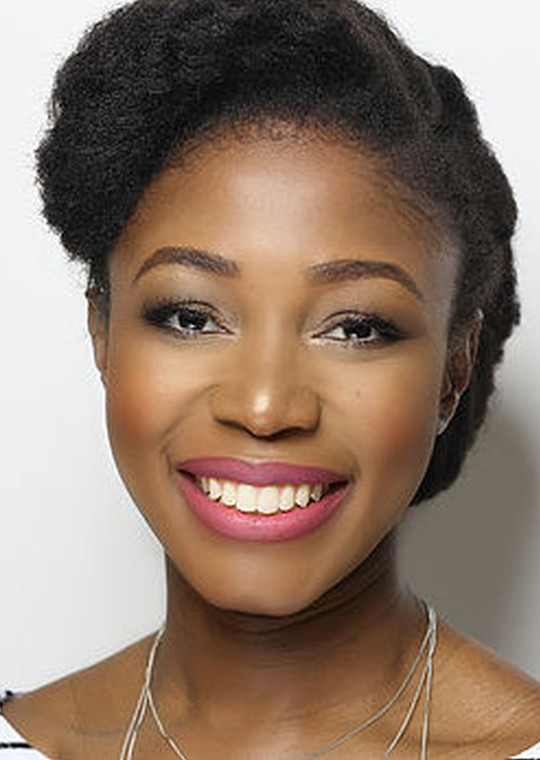
Ana Namburete
Royal Academy Research Fellow based at the Institute of Biomedical Engineering.
Ana
Namburete
IBME, Oxford
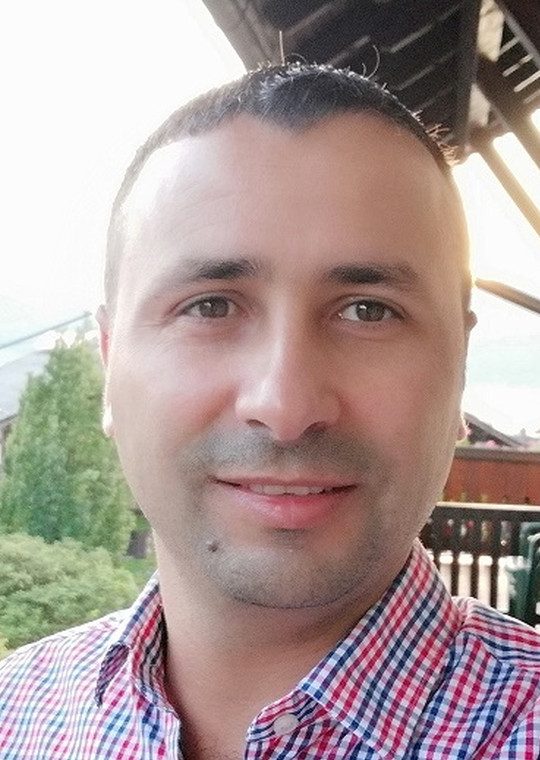
Mohammad Yaqub
Assistant Professor at MBZUAI (Abu Dhabi) and Research Fellow at NDCN (Oxford).
Mohammad Yaqub
MBZUAI, Abu Dhabi and NDCN, Oxford
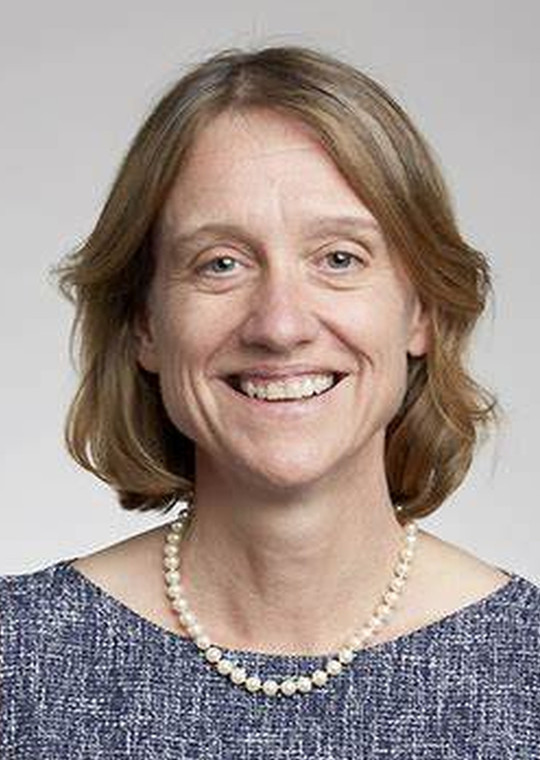
Alison Noble
Technikos Professor of Biomedical Engineering at the Institute of Biomedical Engineering.
Alison
Noble
IBME, Oxford
Contact Us
For further information, please contact us via email and we will get back to you as soon as we can.
Institute of Biomedical Engineering
Old Road Campus Research Building
Roosevelt Drive, Oxford
United Kingdom OX3 7DQ
miua2020@eng.ox.ac.uk
@miua2020ox